Predicting Student's Final Graduation CGPA Using Data Mining and Regression Methods: A Case Study of Kano Informatics Institute
5 (471) · $ 7.99 · In stock
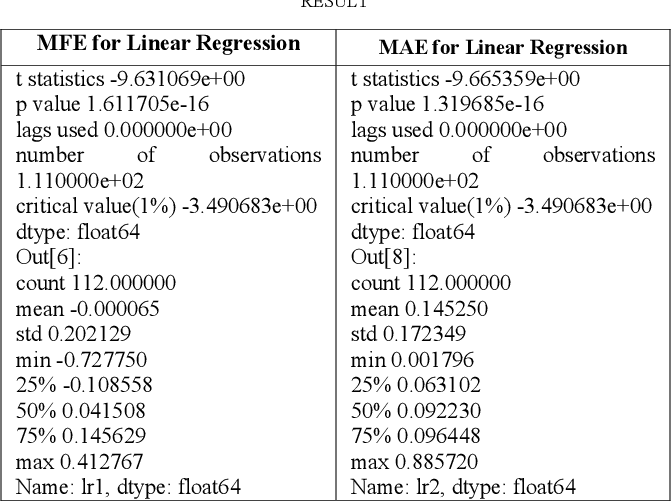
This study uses five regression techniques to analyse students’ first-year cumulative grade point average (CGPA) and predict their final graduation CGPA and linear regression is the model with the mean closest to zero that best fits the data. Data mining and regression techniques are important methods that we can use to predict students’ performance to inform decision making. This study uses five regression techniques to analyse students’ first-year cumulative grade point average (CGPA) and predict their final graduation CGPA. The data set used in this study is that of programming and software development students at Kano Informatics Institute. The results and the grades obtained by 163 students forms the sample data used in the study. The forecast error, mean forecast error and mean absolute forecast error are all calculated. Dickey–Fuller’s stationary t-test is performed for all the regressions analysis values using the Python programming language to determine the mean and if the data is centred on the mean. We use the stationary t-test to test the null and alternative Dickey–Fuller’s hypotheses to compare our P-values and critical values for all regressions analyses done. The results show that the P-values obtained for all the regressions are small and less than the critical value. However, linear regression is the model with the mean closest to zero, and, according to Dickey–Fuller’s statistics, it is the model that best fits our data.

Predicting Student's Final Graduation CGPA Using Data Mining and

Artificial Neural Network with Learning Analytics for Student
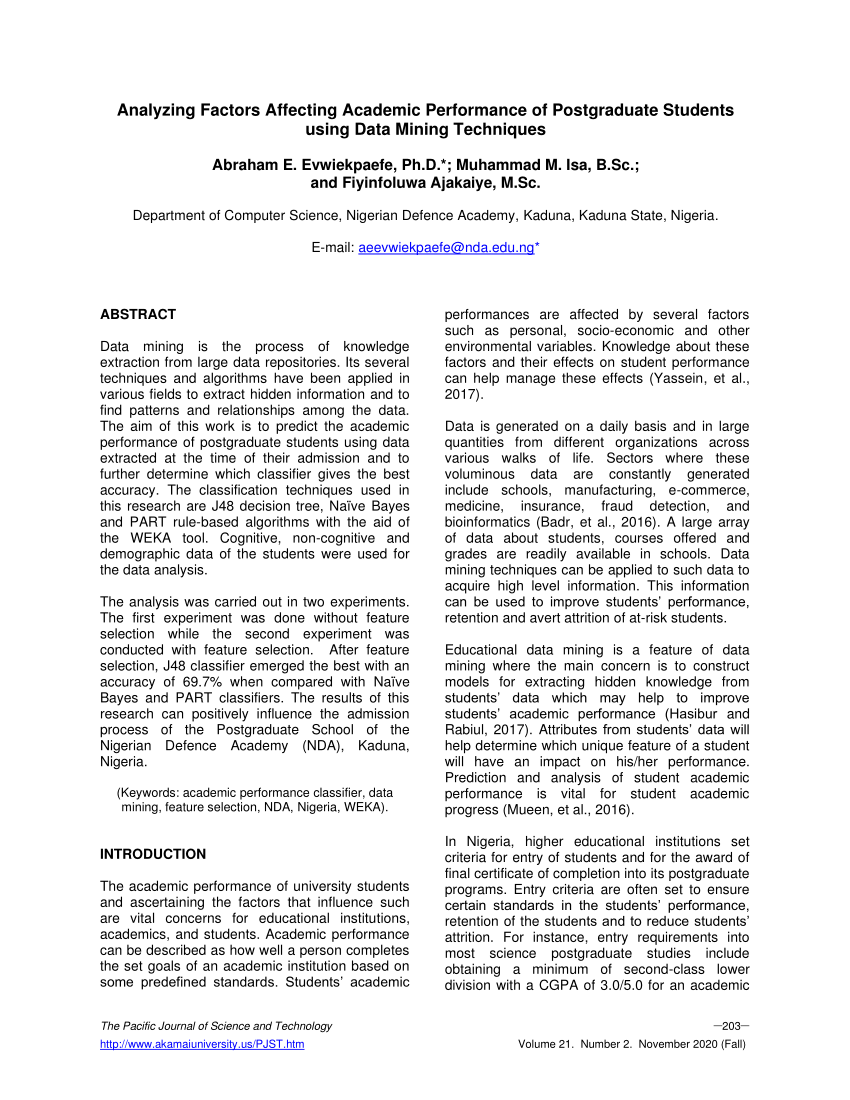
PDF) Analyzing Factors Affecting Academic Performance of

A survey - data mining frameworks in credit card processing
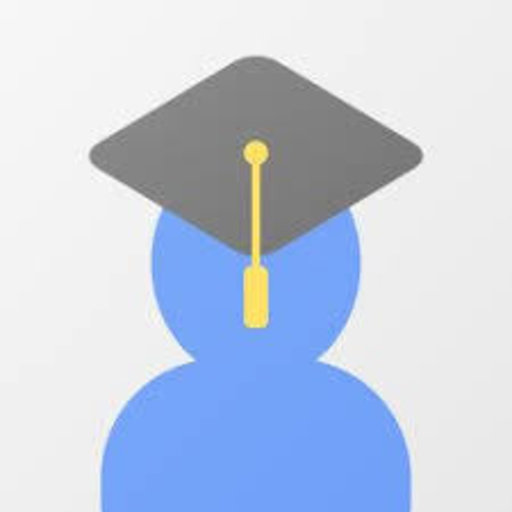
Salim DANBATTA, Lecturer, Ph.D.

Predicting Students' Academic Performance Using Artificial Neural

Early Prediction of Student Success: Mining Students Enrolment Data

Student count by geopolitical zones (test data)

Pictorial Representation of the entire process
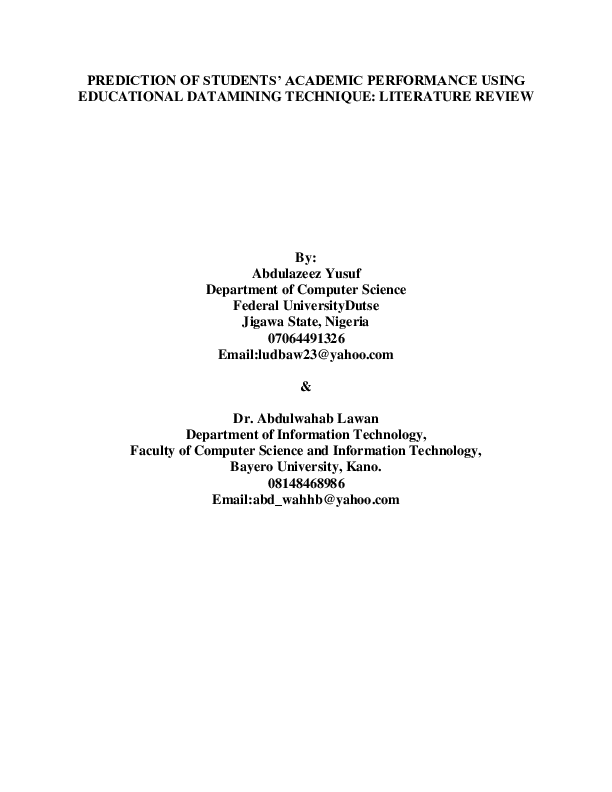
PDF) PREDICTION OF STUDENTS' ACADEMIC PERFORMANCE USING
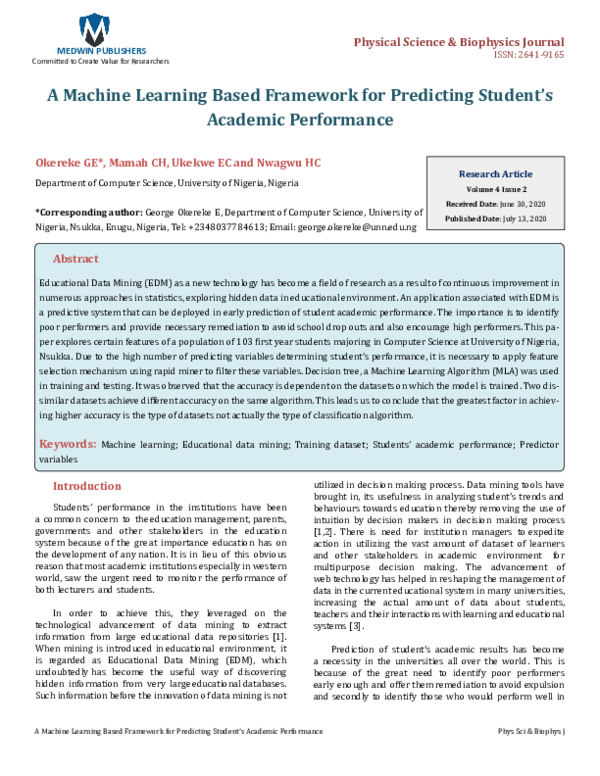
PDF) PREDICTION OF STUDENTS' ACADEMIC PERFORMANCE USING

Predicting Student Academic Performance Using Data Mining
Improved Decision Tree Method in E-Learning System for Predictive Student Performance System During COVID 19